Rabbit, inc. a Los Angeles-based startup, shook the tech world with the launch of their first device prototype, the R1. If you haven’t watched the keynote, it is about 25 minutes long and it is worth it.
The reactions on X are largely positive but there is a healthy dose of skepticism too, as you would expect. Some people feel they MUST have one, while others simply don’t see the use in it. The company sold 10,000 units in the first 24 hours after the launch. You be the judge.
But, instead of discussing the pros and cons of the R1, what I found far more interesting about the keynote is the novel idea of the Large Action Model (LAM), a concept that appears to have been introduced to the world in that keynote, and is the secret sauce of the device and the company.
In this article, with the help of LLMs, we’ll explore the specifics of LAMs and LLMs, understand their differences, and examine how they can be used in parallel to revolutionize various how the business landscape, in layman’s terms.
Let’s begin with a deeper look at Rabbit, inc to understand their role in all of this.
Table of Contents
Background of Rabbit, Inc.
Rabbit,inc, founded in 2022 in Santa Monica, California, is led by a team of experienced AI researchers and entrepreneurs, including Max Levchin (co-founder of PayPal), Jeremy Elman (founder of Coursera), and Ali Mese (formerly of Google AI). Their collective expertise in AI, product development, and business leadership positions Rabbit at the forefront of LAM research and development.
We have developed a system that can infer and model human actions on computer applications, perform the actions reliably and quickly, and is well-suited for deployment in various AI assistants and operating systems. Our system is called the Large Action Model (LAM).
rabbit.tech/research
The core of Rabbit’s technology lies in its LAMs, trained on massive datasets of human-computer interactions. These models can interpret user goals, identify relevant actions within apps, and perform them through programmatic manipulation of interfaces.
Currently, Rabbit’s main product is the R1, a device that can understand natural language instructions, navigate different apps and websites, and complete tasks like booking travel or scheduling meetings.
This opens up possibilities for versatile AI assistants that can adapt to various software environments.
So what exactly is a LAM?
Quick Read: How to Use AI in Marketing: Best Practices 2024!
Large Action Models (LAMs): Bridging Intent and Action
At the heart of LAMs lies their core focus: action execution.
These sophisticated AI models are designed to comprehend user intent and transform it into executable actions across a plethora of applications and interfaces.
This ability makes LAMs incredibly versatile, as they can interact with a wide range of apps, even those without native support.
Their adaptability is another hallmark, allowing them to learn new tasks and adjust to changes in application designs seamlessly.
Furthermore, LAMs support natural language interaction, offering a user-friendly experience.
However, LAMs do have their limitations. They are currently in an early stage of development, which means their real-world applications are somewhat limited. Additionally, they require an advanced infrastructure and a deep understanding of app interfaces.
There’s also the risk of misrepresentation, where a LAM might misinterpret user intent or execute incorrect actions if not trained adequately.
ClickFunnels: A Complete Review!
Large Language Models (LLMs): Masters of Language and Reasoning
LLMs, on the other hand, excel in language processing. Their proficiency in generating and understanding complex texts, including code and poetry, is unmatched.
These models are adept at information retrieval, capable of answering questions by mining and summarizing vast datasets. They also display a basic level of reasoning and abstraction, understanding relationships between concepts.
However, LLMs are not without their own set of challenges. They can perpetuate biases present in their training data and may generate inaccurate information.
Their understanding is often limited to short-term context, struggling with tasks that require real-world understanding or long-term memory.
Additionally, the black-box nature of these models makes it difficult to decipher how they arrive at certain outputs, complicating debugging and error correction.
LAMs and LLMs in Parallel: A Symphony of AI Capabilities
The true potential of LAMs and LLMs lies in their complementary nature. While LLMs focus on language understanding and generation, LAMs excel in executing user commands within applications. This synergy can be harnessed in various consumer and business applications.
- Customer Service Automation: LLMs can understand and process customer queries, while LAMs can take direct actions based on those queries, such as updating records or processing transactions.
- Content Creation and Data Entry: LLMs can generate creative content or structured data, which LAMs can then use to update websites, databases, or even create visual presentations.
- Marketing and Sales Support: LLMs can analyze customer feedback and generate reports, while LAMs can utilize this information to optimize marketing strategies or automate sales processes.
- Business Process Optimization: LAMs can automate routine tasks, while LLMs can analyze and optimize these processes based on historical data and trends.
- Research and Development: LLMs can assist in aggregating and summarizing research materials, whereas LAMs can aid in the practical application of this research in development environments, automating tasks like coding or simulating models.
Integration and Innovation
The integration of LAMs and LLMs represents a significant leap forward in AI applications.
For instance, in a customer service setting, an LLM could comprehend and summarize a customer’s request, while a LAM could execute the necessary actions to resolve the issue, such as scheduling appointments or processing refunds.
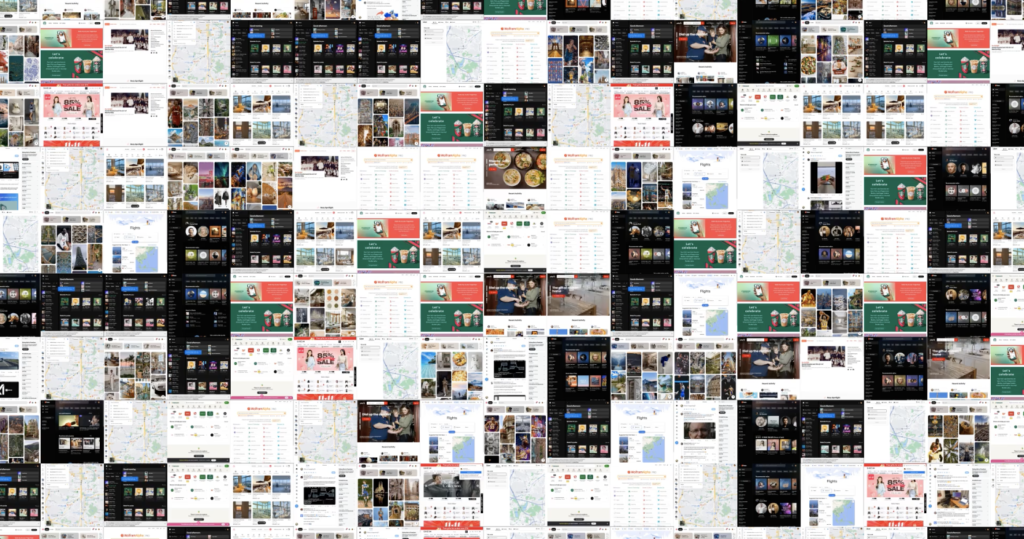
Similarly, in content creation, an LLM might generate a draft article, and a LAM could subsequently format it, insert relevant images, and publish it on various platforms.
In business environments, these models can be transformative.
Imagine a scenario where an LLM analyzes market trends and generates strategic insights, while a LAM implements these strategies in real-time across digital platforms, adjusting marketing campaigns or inventory levels.
Conclusion: A New Step Forward in the AI Revolution
Large Action Models and Large Language Models are not just technological advancements; they are catalysts for change in the digital world.
The potential applications of LAMs are vast, reaching beyond personal assistants into business process automation, customer service, and software development.
Their combined use in consumer and business applications can lead to more efficient, personalized, and intelligent systems.